Today, there are powerful tools that are deployed against the networks of organizations with one goal in mind: to infiltrate those networks and retrieve sensitive and important data. These potential threats carry significant danger to the security systems that are targeted, the sensitive information in question and a company’s reputation, which is harmed if they are unable to keep the customer’s data secure.
This level of cyber threat has brought to the forefront the role of AI and machine learning algorithms in the cybersecurity space. Identifying suspicious activity and responding to it on time is such a large and complex task that AI is now being used to reduce the need for manual threat surveillance, which is not sufficient in today’s cybersecurity world.
What is Malware and Malicious Activity?
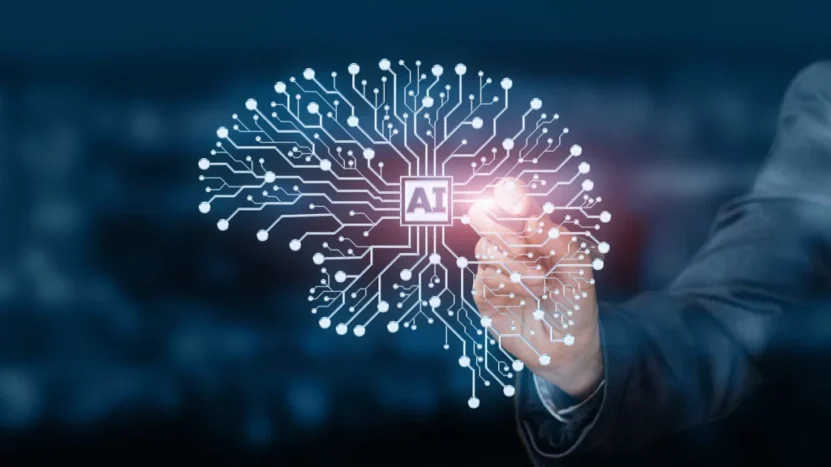
Malware is software that is purpose-built to disrupt, destroy, damage, or gain unauthorized access to a computer system. To be considered malware, they must be involved in what is considered “malicious activity” which includes network attacks, data theft, phishing attacks, viruses, network scanning, ransomware, network disabling, and the attempted or successful removal of security systems, etc.
Why is it Important to Protect from Malware?
Company networks are vital to their core operations and their information security. For many organizations, their data is the most valuable asset their company has, and their business models are built around it. Other companies are equally protective of their data because it is what allows them to operate effectively and provide the type of experience their customers expect.
Why are Artificial Intelligence (AI) and Machine Learning (ML) Crucial for Malware Detection?
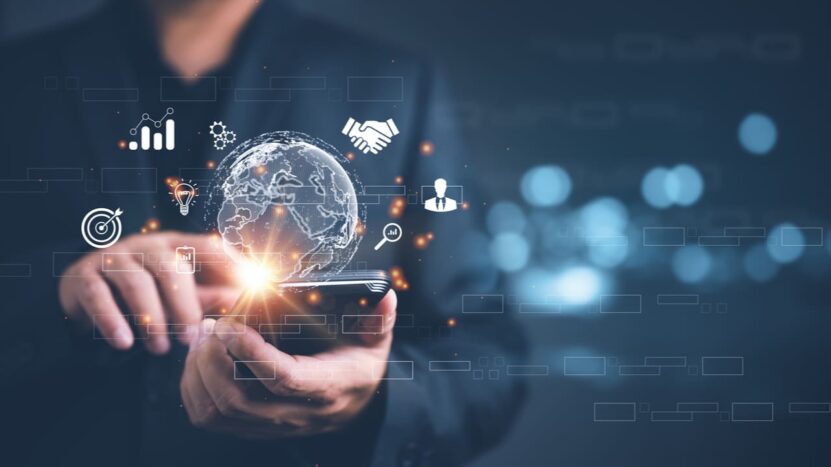
-
AI Models Normal Network Activity, allowing it to See Suspicious Activity
AI cybersecurity programs can detect abnormalities by modelling “normal” network activity and highlighting potential threats through the detection of unusual activity in the network. Armed with this information, AI programs will notify the proper human analysts of the threat, ideally stopping a threat before it has a chance to cause damage.
-
Anomaly Detection Enables Faster “Detect and Respond” Times
Unaided human cybersecurity analysts are never going to be as effective as AI-powered security programs because they cannot sift through large swaths of data at the same rate. By using AI and machine learning to detect anomalies, threat detection has become faster, reducing the damage caused by malicious actors and malware.
According to NTT combining AI and machine learning, can also help prevent future attacks. “These can include basic suggestions to isolate certain systems or more sophisticated directions on how to eliminate threats, going as far as recovering from a potential cyberbreach.”
-
AI and Machine Learning Networks Learn to Identify Patterns
Patterns in data are significant because of the consistent nature of most network behaviour. The regularity of these patterns,which can only be detected when analysing large amounts of data, is one of the most accurate ways to identify threats to a cybersecurity system. How do AI and machine learning programs enhance the ability to detect malicious activity?
- Malware that has been deployed and defeated by other systems has its “signature” analysed and can be used as a comparison tool. Similar to how a human criminal has identifying marks like tattoos or scars, these signatures make any previously battled malware easy to spot and neutralize.
- Another feature that makes AI and machine learning a valuable tool in identifying these patterns is how they can detect specific activities that would flag a program as potentially malicious. What kind of activity? Accessing sensitive data, communicating with unusual parts of a system, such as a command-and-control servers, and if they attempt to modify system files are all examples of activity that would send up flags of concern.
- Finally, the automated classification of these programs as safe or potentially threatening and the ensuing notification of the proper personnel (or the activation of an automated response) is the final step in identifying patterns that can result in network harm.
- Machine Learning Programs Become Better Over Time, Reducing False Positives
No system is perfect and AI-powered systems will inevitably create false positives, which are the innocent, non-malicious activity that has been flagged as potentially dangerous. The beauty of a system that can learn from its mistakes is that as the system matures, it becomes better and better at providing accurate results, reducing false positives, and further enhancing efficiency.
False positives (the flagging of a potential threat that turns out to be a benign program) are one way of continually teaching the system how to better refine its results. While false positives may be time-consuming to inspect, once they are corrected and used as data points that add to the collective knowledge of the system, they add to the overall power of the threat detection system.
-
Real-Time Analysis Provides Real-Time Insights into Threats
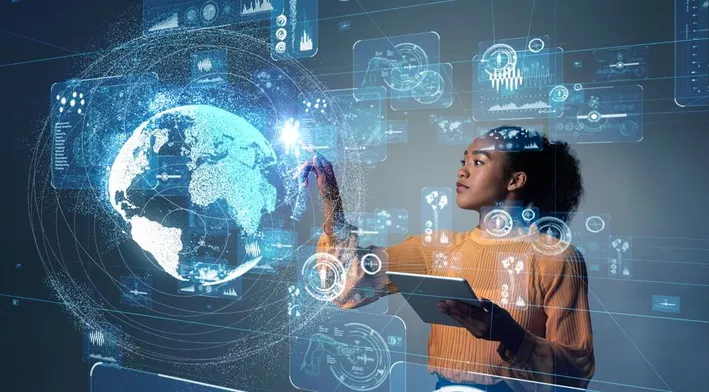
The volume of data that most companies create today would be unmanageable if it weren’t for the assistance of automated security programs. The real-time analysis they provide gives real-time insight into the strengths and vulnerabilities of a given system, which is invaluable to a cybersecurity team.
There is a direct correlation between the time it takes to detect a breach, the time it takes to contain a breach and the financial ramifications of these system failures. Early threat detection is the best way to minimize the cost and the risk of cybersecurity threats. Real-time analysis, powered by the computing strength of an AI-powered system is much better at detecting malicious threats and activity than the traditional security solutions that have dominated the industry.
-
Cost Savings Allow for Better Investment of Resources
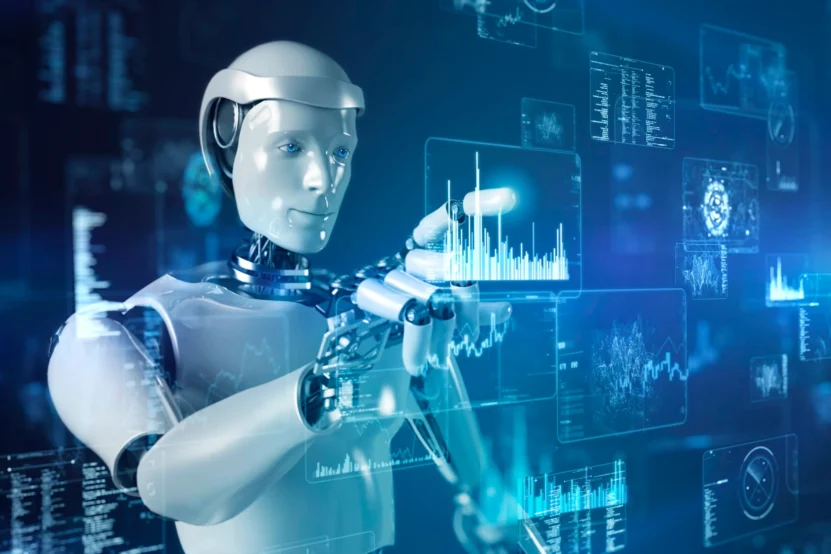
While not directly related to the practical effectiveness of AI and machine learning to detect malicious activity, the potential cost savings of leveraging these systems cannot be overlooked. According to Cybersecuritydive.com, global spending on cybersecurity will top $219 billion in 2024, and it is expected to increase by over 35% by 2026. By using more efficient methods, cost savings can be reinvested into other cybersecurity initiatives, further strengthening a network’s defence.
AI and Machine Learning are Powerful Tools in the Fight Against Malicious Activity
The threat of attack will always be present as malicious actors plot and plan to extract the valuable information companies and organizations have at the heart of their operations. AI and machine learning tools are crucial to the protection of this information, creating a safer marketplace for consumers and businesses alike.
If you want to learn more about how helpful AI is in cyber security, you can always take cyber security courses available online.